Modeling Risk: Insights into Effective Assessment
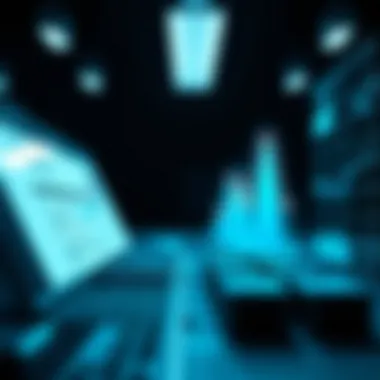
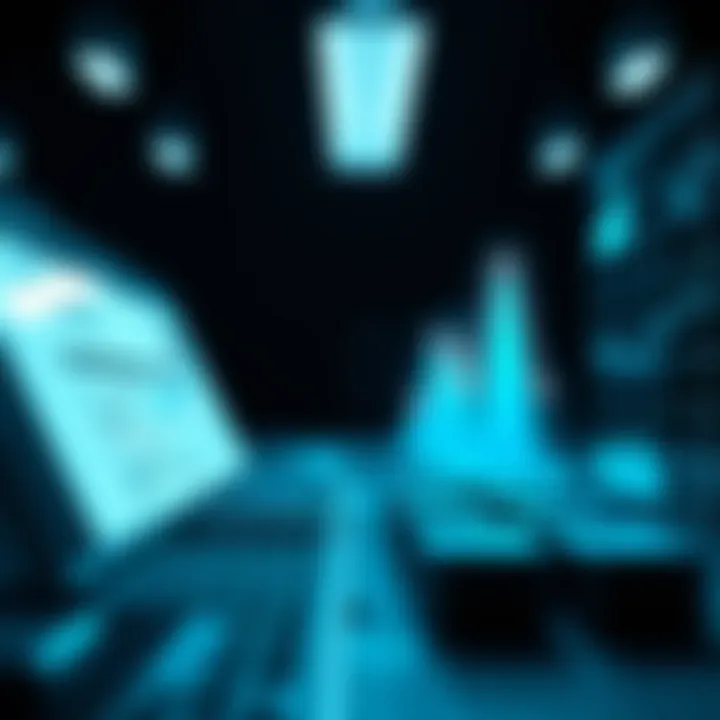
Intro
Risk modeling stands as a cornerstone in the realm of finance, encapsulating a variety of approaches employed by investors and institutions alike. The modern financial landscape, characterized by its volatility and unpredictability, makes it essential for stakeholders to have a clear grasp of risk modeling concepts and tools. Through this exploration, we aim to dissect key methodologies that form the bedrock of effective risk assessment and management.
Definition of Terms
Key Investment Terms Explained
Understanding specific terms in the finance sector can sometimes feel like trying to crack a code. Here are a few key terms to get you familiar with the language of risk modeling:
- Risk: This is the potential for loss or negative outcomes in an investment. It's not merely about uncertainty but about the ways this uncertainty can materialize financially.
- Volatility: This refers to the degree of variation in investment returns. Think of it as the financial roller coaster ā the more unpredictable the ride, the higher the volatility.
- Quantitative Model: A statistical method that uses numerical data to derive risk factors. A good example is Value at Risk (VaR), which estimates potential losses in a portfolio.
- Qualitative Model: This approach relies on descriptive data and judgment rather than numerical analysis. It might involve expert assessments or scenario analysis.
Financial Jargon and Their Meanings
Familiarity with financial jargon can provide a clearer pathway into the world of risk modeling. Hereās a small glossary of popular terms:
- Liquidity: The ease with which an asset can be converted into cash.
- Derivatives: Contracts whose value is derived from an underlying asset. Examples include options and futures.
- Hedging: A risk management strategy that involves making an investment to offset potential losses in another investment.
- Portfolio Diversification: A strategy to spread investments across various assets to balance risk.
Importance in Investing
Why Understanding Terms is Crucial
Grasping the vocabulary of risk modeling is like having a compass in the wilderness. It allows investors to navigate their financial journeys with more confidence. A small misinterpretation of terminology can lead to significant misjudgments in investment decisions.
For example, understanding liquidity can dictate whether an investor should liquidate an asset during a downturn or hold onto it for potential long-term gains. Without this comprehension, an investor might panic-sell and incur losses when a prudent hold might have been the best choice.
How Terminology Impacts Investment Decisions
The relationships among different terms can often affect decision-making processes. Hereās how:
- Volatility and Strategy: Knowledge about volatility can compel an investor to choose defensive strategies during uncertain times.
- Hedging Techniques: If one is well-versed in derivatives terminology, they can better utilize hedging, substantially reducing risk associated with unexpected market shifts.
- Portfolio Management: A thorough understanding of diversification and its associated terms can lead to more balanced portfolios, helping investors to weather market storms.
"Knowledge of terms is not just an academic exercise; it is the bedrock upon which sound investment strategies are built."
In navigating the complexities of risk modeling and its implications, investors who have a strong grasp of these definitions are not only more informed but also well-positioned to exploit opportunities within financial markets.
The continual evolution of risk assessment techniques demands that investors remain vigilant and adaptable. Resources such as Wikipedia, Britannica, and various financial forums can aid in expanding oneās understanding further.
Intro to Risk in Financial Models
In the realm of finance, the intricate dynamics of risk can't be overlooked. Risk modeling is a fundamental cornerstone that shapes decision-making processes, impacts investment strategies, and guides the development of financial products. Understanding risk is not just an academic exercise; it's a critical discipline that empowers investors, regulators, and financial institutions to navigate the tumultuous waters of economic uncertainty.
Defining Risk in Financial Terms
Risk, in a financial context, can be understood as the possibility of an unforeseen event that could lead to financial loss. This could be anything from market fluctuations, credit defaults, to operational failures. In essence, risk is a multifaceted concept that manifests in various forms.
- Market Risk: The potential loss arising from movements in market prices.
- Credit Risk: The risk that a borrower will default on any type of debt.
- Liquidity Risk: The risk of being unable to buy or sell assets quickly enough to prevent or minimize a loss.
To quantify these risks, several metrics and models are employed. For instance, tools like Value at Risk (VaR) provide a statistical measurement of the level of financial risk within a firm or portfolio over a specific time frame.
Understanding these concepts can seem daunting, yet itās crucial for ensuring the sustainability of financial assets in unpredictable markets. Adequately defining and addressing the different types of risk sets the stage for effective risk management.
Importance of Risk Assessment
Risk assessment might just be the most important ingredient in the financial recipe for success. For both seasoned investors and newcomers, assessing risk helps create a clear picture of potential gains against inevitable losses. Here are some key aspects:
- Informed Decision-Making: A thorough risk assessment arms investors with the necessary insights to make informed decisions, helping them weigh the potential rewards against the risks involved.
- Preserving Capital: By understanding where risks lie, investors can design strategies to protect their capital, ensuring long-term financial health.
- Enhancing Strategic Planning: Financial modeling anointed with robust risk assessments allows organizations to forecast and prepare for adverse conditions before they strike.
In finance, taking risks is like casting a fishing line into unpredictable waters. You never know what you might reel in, but a sound strategy increases your chances of success.
Ultimately, thorough risk assessment is not merely a process; itās a strategic backbone that lays the groundwork for every financial endeavor. As we delve deeper into modeling approaches, keep in mind that the importance of understanding risk cannot be overstated.
Understanding Risk Modeling Approaches
Understanding the various approaches to risk modeling is fundamental in grasping how risks are assessed in financial contexts. This section sheds light on different methodologies, including quantitative, qualitative, and hybrid models. Each approach offers unique perspectives and tools that help in navigating the complexities of financial decision-making.
The importance of these methodologies cannot be overstated, as they each have their distinct advantages and considerations. Employing the right model can make all the difference in accurately picturing potential risks, thereby guiding sound investment strategies and complying with regulatory requirements.
Quantitative Risk Modeling
Quantitative risk modeling extracts information from numerical data, enabling analysts to apply statistical techniques and mathematical frameworks to estimate potential losses. This rigorous approach is characterized by its reliance on historical data and statistical methods to quantify risk.
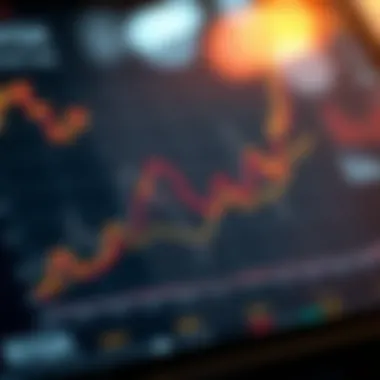
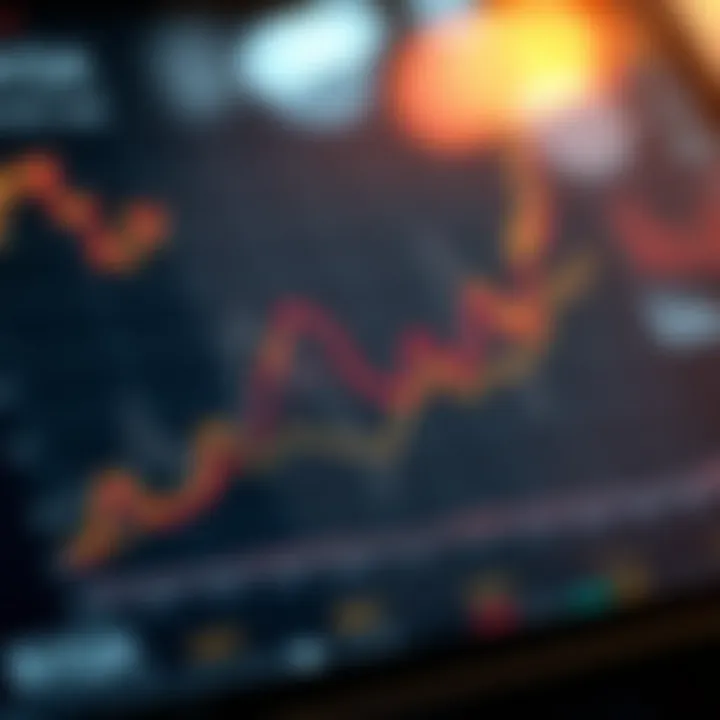
Statistical Methods
Statistical methods serve as the backbone of quantitative risk modeling. This approach not only enables the establishment of predictive frameworks but also enhances decision-making under uncertainty.
- Key characteristics: Statistical methods utilize probability distributions to model risk, providing a systematic way to assess potential outcomes.
- Benefits: One major advantage lies in their objectivity and ability to handle large sets of data, making it a popular choice for analysts. These methods ensure a clearer understanding of the risk landscape, allowing for well-informed decisions.
- Unique features: The use of techniques like regression analysis and hypothesis testing sets statistical methods apart. However, itās essential to note that these models can be sensitive to data quality and may yield misleading results if the underlying assumptions are violated.
Time Series Analysis
Time series analysis involves studying data points collected over time, making it crucial for identifying trends, cycles, and seasonal variations that affect risk.
- Key characteristics: This method focuses on temporal patterns, providing insight into how risk evolves over time.
- Benefits: Time series analysis is valued for its capacity to forecast future trends based on past behaviors, making it particularly useful for financial markets where historical patterns often influence future events.
- Unique features: A distinct aspect of this approach is its ability to capture volatility and sudden shifts in markets. Nevertheless, it can be limited by its reliance on past data and may not account for unforeseen changes, rendering some predictions less reliable.
Monte Carlo Simulations
Monte Carlo simulations allow for modeling of complex systems by generating random samples to understand the range of possible outcomes.
- Key characteristics: This approach is characterized by running simulations that consider thousands of scenarios to evaluate risk.
- Benefits: Monte Carlo simulations provide a comprehensive perspective by highlighting not just expected values but also the ranges of possible outcomes, thus enriching the risk assessment process.
- Unique features: A defining feature is its flexibility across various scenarios, from financial modeling to project management. However, it does require significant computational resources and can become computationally expensive when modeling numerous variables.
Qualitative Risk Modeling
Qualitative risk modeling adds depth to risk assessment by utilizing expert opinions and contextual scenarios to analyze risks, complementing the quantitative methods.
Expert Judgments
Expert judgments leverage the knowledge and experience of seasoned professionals to assess risks that may not be easily quantifiable.
- Key characteristics: This approach emphasizes subjective insights and contextual knowledge, making it valuable in uncertain situations.
- Benefits: The advantage of expert judgments lies in their ability to incorporate nuances and qualitative factors that numbers alone can't capture. They can help in articulating risks that might otherwise go unnoticed in purely numerical analyses.
- Unique features: Notably, expert judgments can vary widely, reflecting personal biases. This subjectivity can introduce inconsistencies, making it essential to weigh these insights carefully alongside quantitative data.
Scenario Analysis
Scenario analysis allows analysts to explore how different variables might impact outcomes under varying circumstances.
- Key characteristics: This technique entails creating hypothetical scenarios that reflect possible future states, providing a framework for assessing impacts.
- Benefits: Scenario analysis is useful for stress testing, revealing how certain events could unfold based on market conditions, policies, or other external factors.
- Unique features: Its capability to stimulate creative thinking around potential future events is one of its redeeming qualities. However, the quality of the analysis is heavily dependent on the scenarios constructed, which can sometimes overlook equally valid possibilities.
SWOT Analysis
SWOT analysis identifies strengths, weaknesses, opportunities, and threats, offering a holistic view of the risk landscape.
- Key characteristics: This method is straightforward and versatile, applicable to individuals, groups, or organizations.
- Benefits: The clear format allows stakeholders to dissect risks systematically, fostering sound strategic planning.
- Unique features: A major advantage lies in its simplicityāintegrating various insights without the need for extensive data. However, the analysis can be superficial if not complemented by deeper investigations or quantitative data.
Hybrid Risk Modeling
Hybrid models combine both quantitative and qualitative approaches, capitalizing on the strengths of each to enhance risk assessment.
Integrating Quantitative and Qualitative Methods
Integrating quantitative and qualitative methods brings together numerical and contextual insights for a more well-rounded risk analysis.
- Key characteristics: This approach allows for a comprehensive understanding of risk by melding objective data with subjective insights.
- Benefits: The synergy can lead to a richer analysis that reveals risks that a purely quantitative or qualitative analysis might overlook.
- Unique features: For instance, incorporating expert judgment into quantitative models helps refine predictions, though challenges remain in reconciling differing data types.
Case Studies of Hybrid Models
Exploring existing case studies provides valuable lessons on the effectiveness of hybrid approaches in diverse settings.
- Key characteristics: Each case reveals how different industries implement hybrid modeling for risk assessment.
- Benefits: These case studies emphasize flexibility, illustrating that various combinations can yield effective results across domains ranging from finance to healthcare.
- Unique features: The diversity in applications demonstrates that tailoring models to specific contexts can maximize their utility. Then again, reliance on multiple methodologies can complicate the analysis process, requiring careful management.
Advantages and Disadvantages
Assessing the advantages and disadvantages of hybrid models further enriches our understanding of their effectiveness.
- Key characteristics: Hybrid models offer a nuanced viewpoint, capturing both numerical accuracy and contextual richness.
- Benefits: By addressing limitations of individual methods, they enhance robustness in risk assessment.
- Unique features: However, the complexity involved and the need for thorough integration can pose challenges, making execution more demanding than with single-method approaches.
In sum, understanding the various approaches to risk modeling is pivotal for investors looking to navigate the multifaceted risks present in financial markets. The right methodology not only aids in mitigating risks but also enhances overall investment strategies.
Commonly Used Risk Models
Risk models form the backbone of understanding various financial exposures and potential pitfalls. Their significance cannot be overstated, especially for financial enthusiasts and investors. As we delve into the realm of commonly used risk models, it is crucial to recognize how they help in assessing risk and making informed decisions. This section covers four pivotal models: Value at Risk (VaR), Conditional Value at Risk (CVaR), stress testing, and credit risk models. Each model has unique features and implications that are vital for navigating the complex world of finance.
Value at Risk (VaR)
Value at Risk, often referred to as VaR, is one of the most prevalent measures used in the financial industry to evaluate the potential loss in value of an asset or portfolio over a defined period for a given confidence interval. In essence, VaR quantifies the worst expected loss under normal market conditions over a specific time frame.
For instance, consider a financial institution that calculates a one-day VaR of $1 million at a 95% confidence level. This indicates that there is only a 5% chance that losses will exceed $1 million on any given day. VaR plays an integral role in risk management and has significant implications in capital allocation, limit-setting, and regulatory compliance.
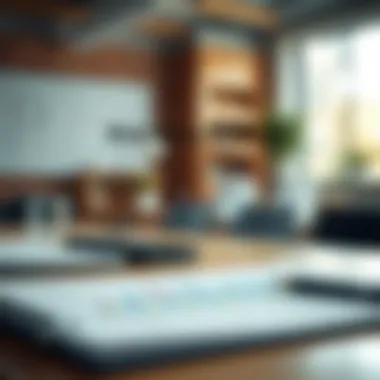
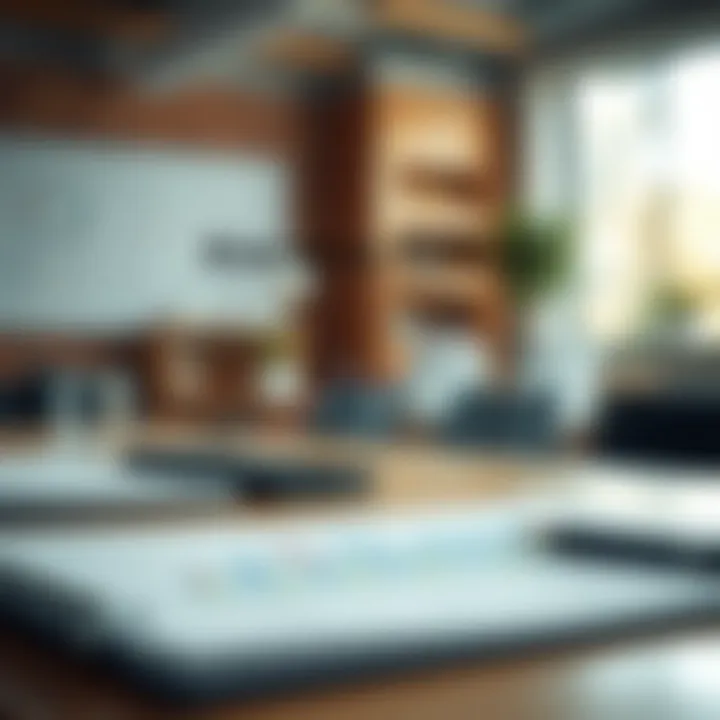
However, it is crucial to recognize VaRās limitations. It does not provide any information about the potential size of losses that exceed the VaR threshold, which can mislead investors if not used alongside other risk assessment tools.
Conditional Value at Risk (CVaR)
Sometimes called Expected Shortfall, Conditional Value at Risk goes a step further than VaR. While VaR indicates the maximum potential loss with a set confidence level, CVaR takes into account the average loss in scenarios where the loss exceeds that value. In other words, it helps in understanding the tail riskāthose severe loss scenarios that VaR might overlook.
For example, if a portfolio's CVaR at a 95% confidence level is $1.5 million, it indicates that, when losses exceed the $1 million VaR threshold, the average loss would be $1.5 million. This model serves as an additional layer of security for risk managers, as it enables better risk mitigation strategies. By focusing on extreme events, CVaR proves to be particularly beneficial during market crises where losses could far surpass expected values.
Stress Testing
Stress testing involves simulating extreme market conditions to evaluate how financial institutions would perform under heightened levels of stress or adverse economic scenarios. It's an essential tool for determining the resilience and robustness of models and portfolios.
As an illustration, during the financial crisis of 2008, many banks used stress testing to analyze their exposure to subprime mortgage assets under considerably deteriorating market conditions. The outcomes often revealed vulnerabilities, prompting proactive adjustments to risk management strategies.
Through stress testing, organizations can identify potential weaknesses in their risk models and ensure they maintain adequate capital buffers. While this process is labor-intensive and often complex, it provides invaluable insights that simple models cannot capture.
Credit Risk Models
Credit risk models are employed to measure the likelihood that a borrower will default on payment obligations. These models are a linchpin within the financial sector as they enable lenders to make informed decisions about credit allocations and assess the potential for loss.
Typically, credit risk models incorporate several factors, including credit scores, financial history, and economic conditions. For example, a bank may use a logistic regression model to quantify the probability of default for a loan applicant. The output can inform lending strategies, interest rates, or even the choice of collateral.
Another widely employed framework is the Z-score model, which evaluates firm performance against bankruptcy risk. Understanding credit risk dynamics is essential, as it shapes lending behaviors and ultimately affects overall economic stability.
"In finance, the shadows of risk loom larger than they seem. Knowing how to model those shadows can illuminate the path ahead."
The Role of Data in Risk Modeling
The significance of data in risk modeling cannot be overstated. In the world of finance, risks are everywhere, lurking in various forms and affecting investment opportunities. Data serves as the backbone for understanding, analyzing, and quantifying these risks. In essence, without reliable data, even the most polished models are like a house of cards: they may look pretty on the surface, but one slight breeze can cause everything to come crashing down. Having accurate and relevant data helps establish a solid foundation for rigorous analysis and informed decision-making.
Consider this: the quality of data directly influences the effectiveness of risk models. High-quality data can reveal patterns, trends, and potential risks that may not be visible at first glance. Data is the critical ingredient that separates insightful analyses from educated guesswork. When evaluating financial risks, the insights provided by data can help investors foresee downturns, avoid pitfalls, and capitalize on opportunities.
By staying attuned to this role, financial enthusiasts can not only enhance their risk models but also improve their investment decision process and outcomes.
Data Sources for Risk Assessment
When it comes to sourcing data for risk assessment, the variety is wide-ranging. Here are several key sources that prove invaluable:
- Market Data: This includes stock prices, commodity prices, and interest rates. For instance, fluctuations in oil prices can affect whole sectors, so keeping tabs on this data is crucial.
- Economic Indicators: Macroeconomic data like GDP growth rates, unemployment rates, and inflation indicators can offer insight into the broader economic landscape. These figures provide context around market behavior.
- Company Financials: Analyzing companies' income statements, balance sheets, and cash flow statements helps investors understand a firmās operational health and potential risks tied to financial stability.
- Historical Data: Studying historical performance under various conditions can guide future expectations. For example, observing how stock market indices reacted during past recessions can illuminate possible future outcomes in similar economic climates.
Utilizing the right mix of these sources can equip investors with valuable insights, thus paving the way for more robust risk models.
Data Quality and Integrity Issues
Data quality and integrity issues can severely undermine the modeling process. Inaccurate, outdated, or incomplete data can lead investors down the wrong pathāmaking decisions on shaky ground. Hereās why paying attention to data quality is essential:
- Inconsistency: Data collected from different sources may not always align standardized formats. Discrepancies in reporting periods, such as fiscal year versus calendar year, can lead to confusion and misinterpretation.
- Timeliness: Using outdated data can be as detrimental as using incorrect data. The financial landscape evolves rapidly; what was true last quarter may not apply today.
- Completeness: Missing data points can skew results. If critical information is left out, it can misrepresent risk levels and lead to misguided decision-making.
Investors should adopt best practices to ensure they are working with high-quality data. Regular audits, cross-referencing data sets, and staying informed about adjustments in data sources can significantly mitigate these issues.
"In the data-rich world, the sharpest knife is the one that cuts through noise and reveals stark truths."
By staying vigilant about data sources and monitoring quality issues, investors can ensure that their risk modeling efforts are not just palatable but genuinely robust and informative. In a whirlpool of numbers and statistics, the right data emerges as a beacon of clarity.
Regulatory Frameworks and Risk Modeling
The regulatory environment plays a crucial role in the landscape of risk modeling. Understanding regulatory frameworks is essential for financial institutions and investors alike, as it provides guidance on best practices and compliance, while also shaping the way risks are assessed and mitigated. These frameworks establish not just the rules of engagement but also influence how models are developed and implemented.
In essence, regulatory frameworks such as the Basel Accords serve as a foundation for ensuring stability in the financial system. They address several important aspects, including capital requirements, risk management practices, and the need for transparency in reporting risk-related information. By adhering to these regulations, financial institutions can manage risk more effectively, which ultimately fosters confidence among investors.
Basel Accords and their Implications
The Basel Accords, developed by the Basel Committee on Banking Supervision, are a set of international banking regulations aimed at ensuring that financial institutions hold enough capital to cover their risks. The three iterations of these accordsāBasel I, Basel II, and Basel IIIāintroduce a range of requirements that significantly impact how banks assess risk.
- Basel I focused primarily on credit risk and laid the groundwork by requiring banks to maintain a minimum capital ratio based on risk-weighted assets and highlighted the importance of maintaining adequate capital reserves.
- Basel II expanded the framework to include operational and market risks, leading to a more comprehensive approach where banks needed to develop internal models for risk assessment. It promoted the idea of "regulatory capital" which takes into account not just the amount of capital but the quality of it as well.
- Basel III, introduced in response to the 2008 financial crisis, places greater emphasis on liquidity and leverage ratios along with capital adequacy, pushing for higher quality capital buffers and better risk management techniques.
The implications of these accords are profound. They compel banks to develop more sophisticated risk models, affecting their lending practices, capital allocation, and approaches to financial stability.
Impact of Regulations on Risk Practices
Regulations don't just impose compliance burdens; they can catalyze substantial shifts in risk management practices. When financial institutions are compelled to comply with these frameworks, it often results in:
- Enhanced Information Sharing: Banks are increasingly required to share and disclose pertinent risk data, which leads to greater transparency and a more informed marketplace.
- Improved Model Validation Processes: Institutions are investing significant efforts in validating and back-testing their models to satisfy regulatory expectations, ultimately resulting in more robust risk assessments.
- Increased Focus on Stress Testing: Regulations like those from the Basel framework mandate rigorous stress testing to evaluate potential risks under adverse conditions. This boosts the overall preparedness of institutions.
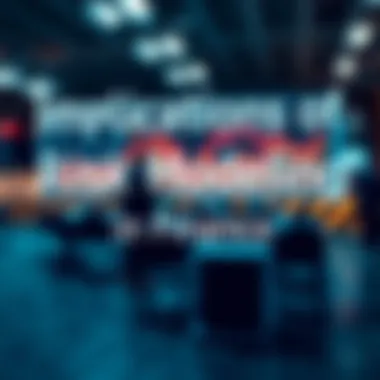
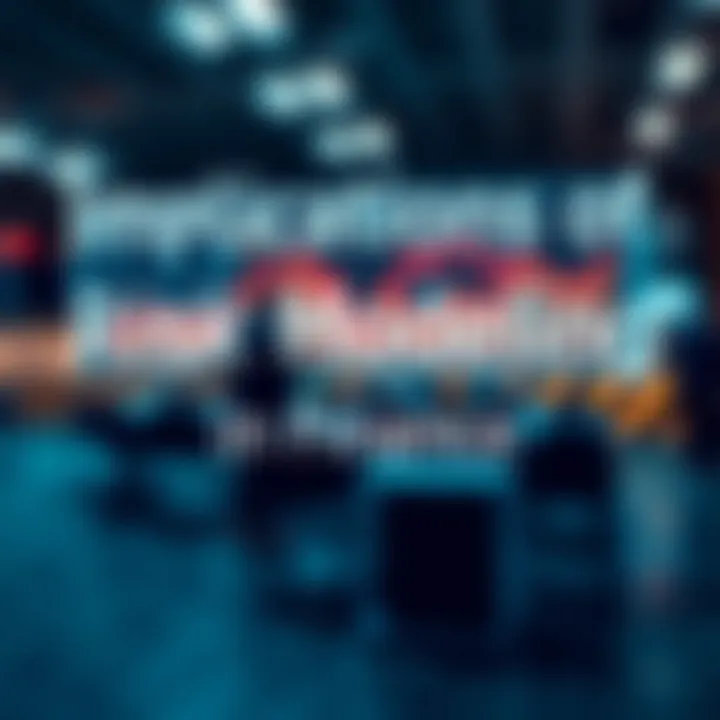
"Good risk management is not just about avoiding failures. It's about seizing opportunities while being aware of what could go wrong."
In summary, the regulatory landscape influences how risk is modeled and assessed in finance. Institutions that adapt to these regulations can not only mitigate risks more effectively but also build trust with investors. As the financial environment evolves, so too must the frameworks that govern risk practices, keeping pace with technological advancements and changing market conditions.
For more information about the Basel Accords, you can visit: Basel Committee on Banking Supervision.
Challenges in Risk Modeling
Risk modeling can be likened to navigating a minefield ā one misstep can send you spiraling into unforeseen troubles. Understanding the challenges inherent in risk modeling is paramount for any financial enthusiast or investor. These challenges shape both the methodology employed and the outcomes derived, ultimately influencing the decision-making process in the realm of investments.
In an era where data drives decisions, the complexities behind risk modeling can often lead to paralysis by analysis. The hurdles faced include model uncertainty and the pitfalls of overfitting or underfitting. Each presents unique obstacles yet both highlight the nuanced nature of tailoring models to best suit the realities of finance.
Model Uncertainty
Model uncertainty arises when there are doubt or variations about the suitability of the model being utilized. This is particularly relevant in risk modeling as numerous variables can affect outcomes, yet predicting these variables accurately is challenging. Essentially, the model chosen could be based on assumptions which, if flawed, lead to inaccurate predictions.
Factors contributing to model uncertainty include:
- Parameter instability: Financial conditions often fluctuate. A model built on past data may not hold true in the future.
- Data quality issues: Relying on poor quality or outdated information can severely impact model accuracy.
- Methodological choices: Selecting the wrong analytical methods or not accounting for critical variables can skew results.
Consequently, model uncertainty necessitates a level of caution. Itās crucial for modelers to perform thorough validations to examine the robustness of their models under different scenarios and data inputs. Heeding this advice can help mitigate risks.
"The greatest enemy of knowledge is not ignorance, it is the illusion of knowledge." ā Daniel J. Boorstin
Overfitting and Underfitting Models
The road to effective risk modeling is riddled with the threats of overfitting and underfitting, two sides of the same coin that can significantly distort findings. Both phenomena lead to ineffective risk assessment that may seem sound at first glance.
- Overfitting occurs when a model is too complex, capturing noise along with the underlying data patterns. Itās like trying to hit a bullseye with a shotgun; you may hit something, but not what you intended. As a result, this model performs admirably with historical datasets but crumbles when faced with new data.
- Underfitting, on the other hand, is when a model is too simplistic to capture the underlying patterns in the data. This leads to an oversimplified view of risk, much like trying to solve a complex puzzle with only a few pieces. Financial conditions are often influenced by intricate, interdependent factors; a simplistic model just doesnāt cut it.
Both scenarios can leave investors feeling like they are standing in front of a locked door, holding a key that doesnāt fit. Therefore, striking the right balance in model complexity is crucial. Regular adjustments based on incoming data and continuous evaluations can keep models aligned with the dynamic nature of financial markets.
In summary, tackling the challenges associated with risk modeling is essential for developing reliable financial insights. By acknowledging the difficulties of model uncertainty and understanding the implications of overfitting and underfitting, investors can create a more resilient framework for decision-making.
Future Trends in Risk Modeling
Risk modeling is continually evolving, necessitating that investors stay abreast of the most prominent trends shaping the landscape. This section will illuminate the significance of future trends in risk modeling, particularly focusing on key elements like technological advancements and new methodologies that have the potential to enhance risk management strategies. In an increasingly interconnected world, recognizing these trends is paramount for making informed investment decisions and for developing strategies that can withstand financial volatility.
Advancements in Technology
Technology plays a crucial role in transforming risk modeling practices. Tools that were once cutting-edge are now becoming standard in the field, emphasizing the importance of staying current with emerging technological solutions. For example, cloud computing is revolutionizing how data is stored and analyzed, allowing for real-time assessments that were previously unfeasible.
Moreover, the increasing capabilities of data analytics tools enable investors to handle vast datasets effortlessly. This can lead to more precise and timely risk assessments.
- Cloud-based Solutions: These allow stakeholders to leverage cutting-edge technology without the hefty equipment price tags. This democratization of technology means that even smaller firms can effectively employ sophisticated risk modeling techniques.
- Robust Data Processing: The ability to analyze extensive and varied data sources fosters a comprehensive view of risk. This comprehensive analysis is essential in identifying potential risks that might not be immediately evident.
- Visual Analytics: With advancing visualization tools, conveying complex risk data becomes clearer, aiding decision-makers in drawing insights and strategies efficiently. Tools like Tableau and Power BI illustrate how data visualization is changing the narrative in risk assessments.
"Staying ahead in risk modeling requires not just technical knowledge but also a keen awareness of emerging technologies and methodologies."
AI and Machine Learning in Risk Assessment
The rise of artificial intelligence and machine learning is arguably one of the most impactful trends in risk assessment. These technologies bring about a paradigm shift, enabling risk managers to identify patterns and anomalies within data that might otherwise go unnoticed. The advantages are multi-fold:
- Predictive Analytics: Machine learning algorithms can forecast market trends based on historical data, thus allowing institutions to take preemptive actions against potential risks. For emotions like fear that drive market volatility, insights generated from AI can buffer against irrational market behaviors.
- Automation of Processes: By automating routine tasks such as data entry, institutions not only save time but also minimize human error. In an environment where every second counts, this efficiency becomes invaluable.
- Enhanced Decision-Making: AI tools can analyze multiple scenarios simultaneously and provide data-driven recommendations swiftly. This is particularly useful in stress testing and when evaluating the potential impact of financial decisions.
The integration of these technologies into processes will be critical as the financial landscape evolves. Those who adeptly harness AI and machine learning stand to gain a significant competitive edge, making these not just trends to watch but vital components of future risk modeling.
Epilogue and Key Takeaways
The conclusion of this exploration into the modeling of risk serves as a vital wrap-up of complex methodologies and their real-world applications. Within this article, we have deciphered various risk modeling techniques, each contributing unique insights into how to interpret and manage financial uncertainties. This synthesis not only reiterates the value of different perspectivesābe it quantitative, qualitative, or hybrid methodsābut also emphasizes the critical need for adaptability in an ever-shifting financial landscape.
Summarizing Risk Modeling Techniques
Each risk modeling technique mentioned throughout this article carries its own weight and significance.
- Quantitative Risk Modeling: This approach relies heavily on statistical tools and data analysis to predict potential financial losses. Models such as Value at Risk help in defining exposure levels, particularly crucial for financial institutions striving to maintain compliance with regulatory thresholds.
- Qualitative Risk Modeling: On the flip side, qualitative methodsāthrough expert judgment and scenario buildingāoffer a narrative context to the numbers. They bring human intuition and experience into the mix, which can be equally essential in risk assessment processes.
- Hybrid Risk Modeling: The blend of quantitative and qualitative approaches allows for a more nuanced analysis. By pairing hard data with soft insights, investors can make decisions grounded in both empirical evidence and experiential wisdom.
Understanding these techniques gives financial enthusiasts a toolkit to better navigate the choppy waters of investments.
Importance of Continuous Learning
In the realm of risk modeling, stagnation equals obsolescence. One cannot overstate the significance of keeping pace with evolving methodologies, emerging technologies, and changing regulatory landscapes. Continuous learning fosters resilience and adaptabilityāqualities indispensable for investors and risk managers alike.
- Engaging with ongoing education, whether through workshops, online courses, or peer mentorship, enables individuals to enhance their understanding of new tools and strategies.
- Staying attuned to industry changes through resources such as inancialeducatorscouncil.org or bankingtech.com helps in grasping how innovations like AI and machine learning are reshaping risk assessment today.
In essence, to thrive in the dynamic world of finance, one must cultivate a mindset oriented toward lifelong learning, a principle that can lead to more informed, confident decision-making in both personal and professional investment strategies.
"Risk comes from not knowing what you're doing." ā Warren Buffett
Thus, as we conclude our discussion, the journey doesnāt end here. Rather, it's merely a foundation on which every reader can build their own strategies in risk modeling and assessment.