Understanding P-Value: A Guide for Investors
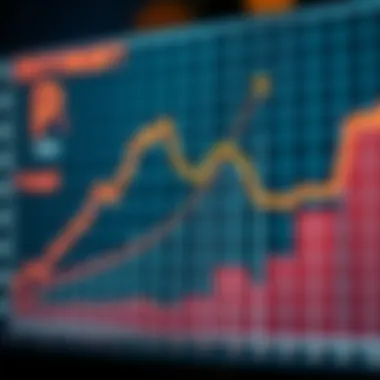
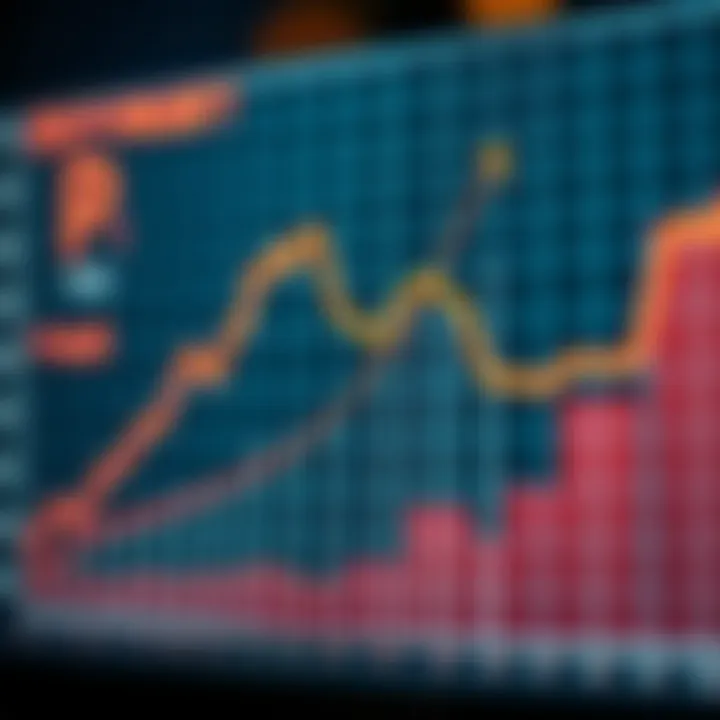
Intro
In the complex world of investing, terminology often feels like a minefield. One such term that frequently pops up, especially in conversations around statistical significance, is the p-value. Although it’s rooted in statistical theory, understanding this concept can offer substantial insight to investors looking to base their decisions on solid evidence rather than sheer speculation.
At its core, the p-value serves as a gauge that helps investors discern whether observed data are consistent with a specific hypothesis. For instance, when analyzing the potential returns of a new technology or strategy, a low p-value might suggest that the results observed are not merely due to random fluctuations.
The world of finance can be fraught with uncertainty, and equipping oneself with a grasp of p-values can sharpen an investor's acumen. This guide endeavors to peel back the layers of the p-value concept, enabling investors to leverage this statistical measure in their strategic planning. So let's dive in!
Definition of Terms
Before we get our feet wet in the significance and calculations surrounding p-values, it’s crucial to grasp some underlying terms. Understanding these definitions can significantly enhance your investment discussions and decision-making.
Key Investment Terms Explained
- Hypothesis: A statement that can be tested. In investing, this might sample that "tech stocks will outperform traditional stocks this quarter."
- Statistical Significance: A measure that indicates whether the results of a study are likely to be true or simply due to chance. It often relies on the p-value.
- Confidence Level: Refers to how sure you can be in the results. A common confidence level is 95%, meaning that you can be 95% certain of your results.
- Null Hypothesis: The default position or starting hypothesis that assumes no effect or no difference. In the context of investment, it might posit that there is no difference in returns between two types of investments.
- Alternative Hypothesis: This directly opposes the null hypothesis. Following the earlier example, it could state that tech stocks will indeed outperform traditional stocks this quarter.
Financial Jargon and Their Meanings
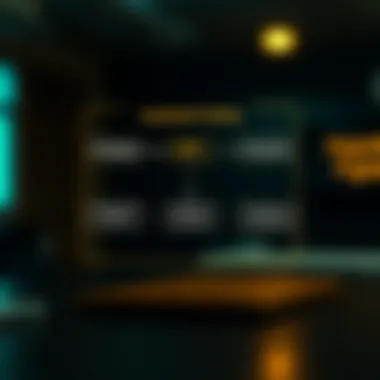
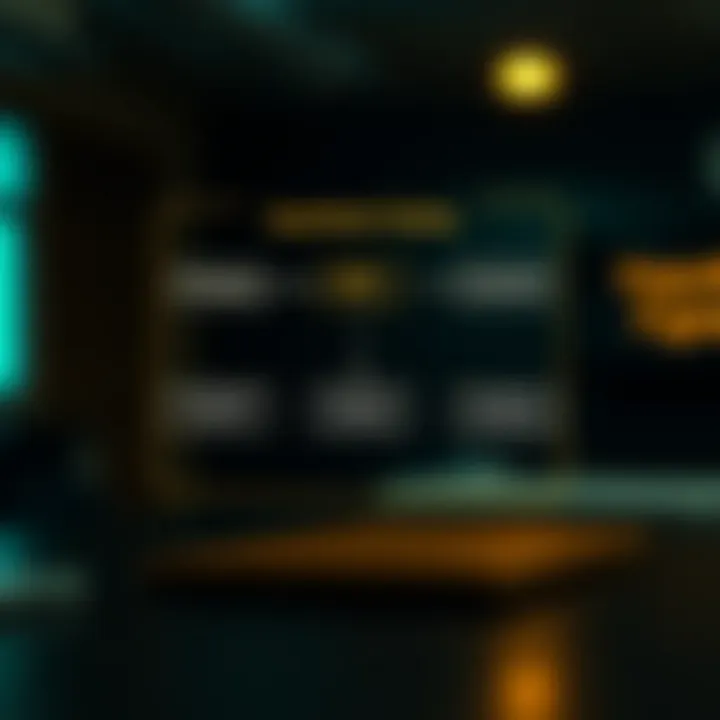
Navigating the jargon of finance can feel like trying to decode a foreign language. Here’s a quick refresher on some terms that could appear alongside discussions about p-values:
- Regression Analysis: A statistical method for estimating the relationships among variables. It often helps in predicting future trends based on historical data.
- Type I Error: Incorrectly rejecting the null hypothesis when it is true. In simpler terms, it’s like crying wolf; you declare a significant difference when there isn’t one.
- Type II Error: Failing to reject the null hypothesis when it is false. This means missing out on a real opportunity.
Understanding these terms lays the groundwork for deeper discussions about p-values and their relevance in financial analysis.
Importance in Investing
Understanding jargon and definitions is just the first step; it cements a foundation for informed decision-making.
Why Understanding Terms is Crucial
Investment decisions are seldom made on gut feelings alone. Familiarity with key terms enriches the dialogue between investors, financial advisors, and even during boardroom discussions. A well-informed investor can challenge assumptions and contribute to risk assessments much more effectively, making for a more dynamic decision-making process.
How Terminology Impacts Investment Decisions
Decisions made on statistical principles can mean the difference between a sound investment or a leap of faith off a cliff. Here’s how language and definitions shape outcomes:
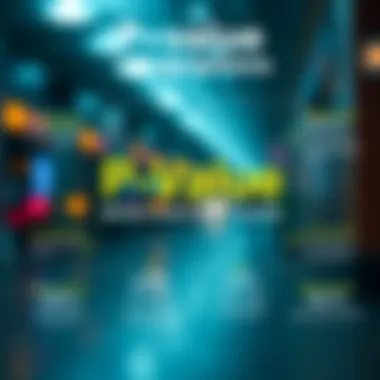
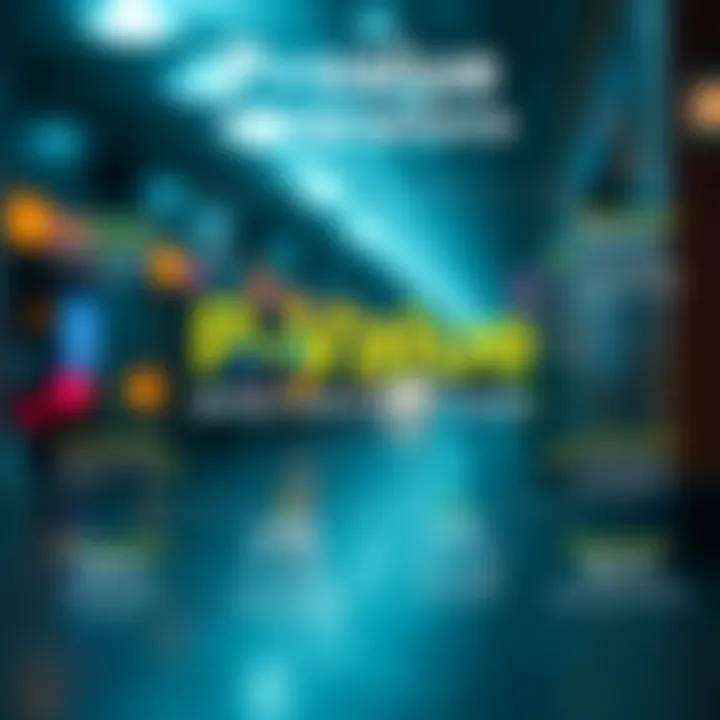
- Informed Risks: With a solid grasp of what a p-value reflects, investors can analyze whether the risks associated with a given investment are justified.
- Strategic Reasoning: Knowledgeable investors often make calculated decisions based on p-values, altering strategies to hedge against potential losses.
- Market Predictions: Investors who can navigate the semantics of data can better predict trends and adjust their portfolios accordingly.
By investing time in understanding p-values and related terminology, you position yourself to make astute choices in an era inundated with data. The next sections of this guide will unravel the workings of p-values in depth, exploring their calculations and practical applications in investment strategies.
"In finance, as in life, the more you know, the better decisions you make."
For additional resources on p-values, consider visiting Wikipedia or Britannica.
Stay tuned as we delve further into the intricacies of how p-values play a pivotal role in financial analyses, enhancing both knowledge and decision-making power.
Foreword to P-Value
Understanding the concept of p-value sits at the intersection of statistics and investment analysis. For those who navigate the fluctuating waters of financial markets, having a solid grip on statistical measures such as p-value can be as crucial as knowing market trends. This section aims to illuminate the idea of p-value, clarifying its importance and practical implications within the sphere of investment.
Defining P-Value
At its core, the p-value is a statistical measure that helps to determine the strength of evidence against a null hypothesis. It quantifies the probability of observing results at least as extreme as the ones measured in a study, assuming that the null hypothesis is true. More simply put, if you find a very small p-value, it suggests that what you’re observing is unlikely to occur under the assumption that the null hypothesis holds.
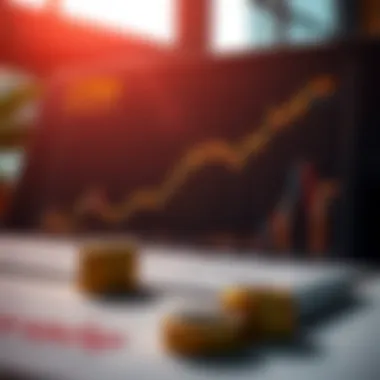
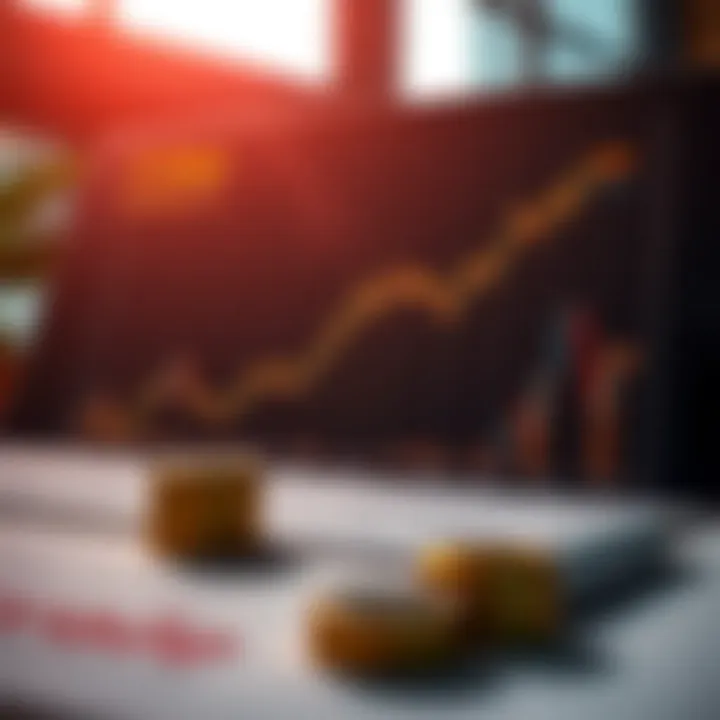
To put it in real-world terms, imagine you're contemplating whether a new investment strategy outperforms an established one. The p-value gives you a numerical way to assess how confident you should be in that conclusion. A p-value below a common threshold, like 0.05, has generally become a key indicator that the evidence you’re observing is significant enough to reject the null hypothesis, steering your investment decisions accordingly.
Historical Context
The journey of p-value's acceptance into the statistical toolkit is as compelling as the measure itself. Its roots can be traced back to the early 20th century, notably attributed to the renowned statistician Ronald A. Fisher. In 1925, Fisher introduced the idea of the p-value in his work on statistical methods for research in agriculture. Through time, this notion progressively became integral in research and analysis within various scientific fields, including social sciences and finance, among others.
Through the years, the p-value has faced scrutiny and skepticism. Some skeptics argue that reliance on a singular threshold oversimplifies the complexity inherent in data interpretation. Still, its legacy remains cemented as a foundational element of hypothesis testing. Understanding this historical backdrop helps investors not only appreciate the significance of p-values but also recognize the evolution of statistical reasoning in investment decision-making.
This initial dive into p-value establishes a platform from which to explore further nuances in the valuation of financial data. Grasping its definition and history enhances investors’ ability to utilize this statistical measure effectively as they assess risks and opportunities in the market.
The Mathematics Behind P-Value
Understanding the mathematics behind the p-value is crucial for investors, as it provides the backbone for making sense of data-driven decisions. This section demystifies the calculations involved, exploring how they are applied within investment strategies and risk assessment scenarios. Grasping these mathematical principles not only hones analytical skills but also enhances a person’s ability to read between the lines of statistical outcomes, leading to informed investment choices.
Formulaic Representation
The formula for calculating a p-value varies depending on the statistical test being employed. Generally, it stems from the observed data and reflects the probability of obtaining a test statistic at least as extreme as the one observed, assuming the null hypothesis holds true.
For a simple understanding, when dealing with hypothesis tests, the p-value can be expressed as:
plaintext p-value = P(X ≥ x | H0)